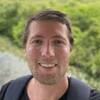
How Hugging Face Grew to a $4.5B Valuation in Seven Years
Who is Clément Delangue?
Clement Delangue is the co-founder and CEO of Hugging Face, a company revolutionizing the AI and natural language processing space. Born in La Bassée, France, Delangue began his career in the product team of Moodstocks, a machine learning startup later acquired by Google. He holds a degree from ESCP Business School and has taken computer science courses through Stanford's Engineering Everywhere program.
What problem does Hugging Face solve?
Hugging Face solves the problem of making powerful machine learning models accessible by offering a vast library of open-source resources, allowing developers to easily implement advanced AI without prohibitive costs or expertise, thus enabling wider innovation and efficiency in AI applications.
How did Clément come up with the idea for Hugging Face?
The idea for Hugging Face began when Clément Delangue and his co-founders envisioned creating a chatbot app designed to engage and provide emotional support to teenagers. As they delved deeper into this undertaking, they quickly encountered the limitations of existing technologies, which ignited their interest in harnessing the potential of open-source machine learning models to enhance their chatbot's capabilities. This pivot led them to embrace and contribute to the burgeoning field of natural language processing (NLP) through open-source frameworks.
The release of Google's influential "Attention Is All You Need" paper in 2017, introducing Transformers as a groundbreaking technology, was a major catalyst for Hugging Face. Recognizing the transformative potential of Transformers, the team realized the need to make these powerful tools more accessible to developers and researchers worldwide. Their efforts to port and open-source the BERT model from TensorFlow to PyTorch allowed them to provide an accessible platform that bridged the gap between cutting-edge academic research and practical applications in industry.
Through observing the community's enthusiastic response and adoption of their open-source library, Delangue and his team were able to validate the shift from a closed chatbot model to a more open, collaborative platform. This journey from ideating a chatbot to becoming a hub for NLP innovation highlighted the importance of community-driven development and the vast potential of Transformers in reshaping AI technology. Hugging Face learned that sharing models openly leads to improvements in technology development and grants enterprises the opportunity to further refine and tailor these models to their specific needs, fostering rapid innovation and democratization in the field of AI.
How did Clément build the initial version of Hugging Face?
Hugging Face began as an AI-powered chatbot application in 2016, designed for emotional support and entertainment for teenagers. Initially utilizing open-source AI models, the company soon recognized the broader potential of these models for Natural Language Processing (NLP) and shifted focus accordingly. In 2018, the founders released their first major open-source library, Hugging Face Transformers, inspired by Google’s BERT model, which offered superior performance via transfer learning. The development process involved porting existing models initially released in TensorFlow to the PyTorch framework to democratize access, which catalyzed the rapid growth of their library. Developing these transformer models entailed significant computational resources, requiring extensive pre-training on large datasets, followed by more efficient fine-tuning specific to task domains. Hugging Face's transition to a community-centric machine learning platform was driven both by the growing demand for flexible AI tools and the technical challenges of managing the complex, large-scale infrastructure necessary for training models.
What were the initial startup costs for Hugging Face?
- Series A Funding: In December 2019, Hugging Face raised $15 million.
- Series B Funding: Hugging Face secured $40 million in March 2021.
- Series C Funding: The company raised $100 million from Sequoia and KOTU.
- Series D Funding: In 2023, Hugging Face raised $235 million, leading to a valuation of $4.5 billion.
How did Clément launch Hugging Face and get initial traction?
Open Source Community Engagement
Hugging Face initially focused on building an open-source community by releasing their natural language processing (NLP) models and tools on GitHub. They started by offering models like BERT in PyTorch, gaining significant traction from developers and researchers interested in these state-of-the-art models.
Why it worked: By providing valuable open-source tools, Hugging Face fostered community collaboration and quickly became a central hub for AI model sharing and development. This approach allowed them to tap directly into the growing interest in transformer models and build a user base organically through collaboration and contributions.
Organic Word-of-Mouth and Social Proof
The broad appeal of their open-source framework led to widespread adoption among researchers and companies. Hugging Face capitalized on this momentum by encouraging users to share their experiences and successes using their tools, effectively allowing the community to vouch for the platform's capabilities.
Why it worked: Harnessing organic word-of-mouth allowed Hugging Face to spread its reach without traditional marketing efforts. The credibility from academic and corporate users showcased the reliability and effectiveness of their tools, further enticing new users to try their platform.
Academic and Research Collaboration
Hugging Face prioritized collaboration with academic researchers and institutions, which helped them keep the platform at the forefront of AI advancements. By supporting various research initiatives and providing an accessible platform for model training and sharing, they enriched their toolset while simultaneously attracting academics.
Why it worked: Engaging directly with the academic community ensured that Hugging Face's tools remained relevant and cutting-edge, attracting ongoing input and improvements from top minds in the field. This strategy solidified their reputation as a trusted and innovative platform for AI research and development.
What was the growth strategy for Hugging Face and how did they scale?
Open Source Community and Platform
Hugging Face has achieved significant growth through its community-driven approach. This platform hosts over a million models, datasets, and applications, allowing researchers, developers, and companies to contribute and collaborate. This strategy has fostered a vibrant community that actively generates and shares various machine learning models, greatly amplifying Hugging Face's reach and impact.
Why it worked: The open-source nature of Hugging Face lowered the barriers to entry, allowing anyone interested in AI and NLP to participate. By positioning itself as the "GitHub for machine learning models," Hugging Face attracted a broad user base, including major tech companies and over 10,000 organizations using its platform. The ability for users to fine-tune pre-trained models for their own needs encouraged widespread adoption, creating a network effect that further bolstered the platform's growth.
Strategic Partnerships and Funding
Hugging Face has secured funding from major tech players like Google, Salesforce, and Nvidia, culminating in a valuation of $4.5 billion following a $235 million Series D round. These partnerships not only provided financial resources but also strategic positions within the industry, allowing Hugging Face to expand its influence and accelerate product development.
Why it worked: Strategic partnerships enhance Hugging Face's credibility and bring in resources that facilitate further innovation and expansion. By aligning with industry giants, the company gains access to expertise and potential collaborations that can improve their product offerings and market reach. This financial backing supports Hugging Face's mission to democratize machine learning while providing the necessary tools and infrastructure for its community.
Product Offering and MLOps Expansion
With products like the Transformers library and the Hugging Face Hub, the company has positioned itself as a crucial tool for machine learning practitioners. Hugging Face also provides an Inference API and AutoML solutions, simplifying the deployment and management of machine learning models. There are indications that Hugging Face may explore the MLOps market more deeply, which could open new revenue streams and solidify its position in AI deployment and management infrastructure.
Why it worked: By offering a range of services that cater to differing levels of expertise—from individual developers to large enterprises—Hugging Face maximizes its user base. Providing easily accessible open-source tools attracts developers, while enterprise-level solutions cater to larger businesses needing more comprehensive support. This dual approach allows Hugging Face to grow its community and revenue concurrently.
Ethical AI and Inclusivity Efforts
Hugging Face has actively worked towards ethical AI by addressing bias in AI models and promoting fairness. They have introduced tools like model cards to help identify potential biases and flaws in models and have conducted community sprints to improve language inclusivity, particularly for low-resource languages.
Why it worked: Being proactive in addressing ethical concerns resonates well with the AI community and builds trust among users. This commitment to ethical AI positions Hugging Face as a responsible leader in the industry, attracting users who value transparency and fairness in technology, thus broadening their appeal even further.
What's the pricing strategy for Hugging Face?
Hugging Face utilizes a freemium pricing model, offering free access to basic features while charging $9/month for a pro plan and providing custom pricing for enterprise solutions, with additional fees for services like APIs and Autotrain.
What were the biggest lessons learned from building Hugging Face?
- Embrace Open Source: Hugging Face thrived by adopting an open-source model, which fostered a strong community and accelerated innovation. This approach not only democratized AI access but also created immense value through community contributions.
- Adapt and Pivot: Initially focused on building a chatbot, Hugging Face pivoted to creating an open AI platform when they observed immense community interest. This flexibility allowed them to scale and meet market demands effectively.
- Prioritize Ethical AI: By openly addressing biases and promoting transparency, Hugging Face underscored the importance of ethical AI development. Introducing initiatives like Model Cards helped users make informed, responsible AI deployments.
- Value Community Contributions: Encouraging community involvement in model and dataset sharing increased their platform's utility and innovation. This approach not only expanded their reach but also leveraged diverse perspectives for continual improvement.
- Persist Through Challenges: The journey included multiple shifts and learning curves, but Hugging Face's persistence, including tackling complex tasks like multilingual transformers, showcased the resilience required to succeed in the fast-evolving AI landscape.
Discover Similar Business Ideas Like Hugging Face
BugRaptors is a quality assurance brand with a team of over 200 ISTQB-certified testers, present in India, the United States, the United Kingdom, and Australia, serving over 10 industry verticals with their own proprietary test automation frameworks and tools, catering to various Fortune 500 brands and SMEs with 30-40% reduced time to market and the confidence of zero-defect leakage for their products or operations.
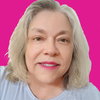
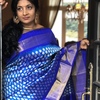
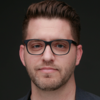
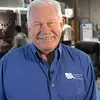
More about Hugging Face:
Who is the owner of Hugging Face?
Clément Delangue is the founder of Hugging Face.
When did Clément Delangue start Hugging Face?
2016
What is Clément Delangue's net worth?
Clément Delangue's business makes an average of $4.2M/month.
How much money has Clément Delangue made from Hugging Face?
Clément Delangue started the business in 2016, and currently makes an average of $50.4M/year.
Download the report and join our email newsletter packed with business ideas and money-making opportunities, backed by real-life case studies.
Download the report and join our email newsletter packed with business ideas and money-making opportunities, backed by real-life case studies.
Download the report and join our email newsletter packed with business ideas and money-making opportunities, backed by real-life case studies.
Download the report and join our email newsletter packed with business ideas and money-making opportunities, backed by real-life case studies.
Download the report and join our email newsletter packed with business ideas and money-making opportunities, backed by real-life case studies.
Download the report and join our email newsletter packed with business ideas and money-making opportunities, backed by real-life case studies.
Download the report and join our email newsletter packed with business ideas and money-making opportunities, backed by real-life case studies.
Download the report and join our email newsletter packed with business ideas and money-making opportunities, backed by real-life case studies.